Table Of Content
Some variables, like temperature, can be objectively measured with scientific instruments. Others may need to be operationalised to turn them into measurable observations. If your study system doesn’t match these criteria, there are other types of research you can use to answer your research question. Then you need to think about possible extraneous and confounding variables and consider how you might control them in your experiment. In this course we will pretty much cover the textbook - all of the concepts and designs included. I think we will have plenty of examples to look at and experience to draw from.
Bayesian Designs
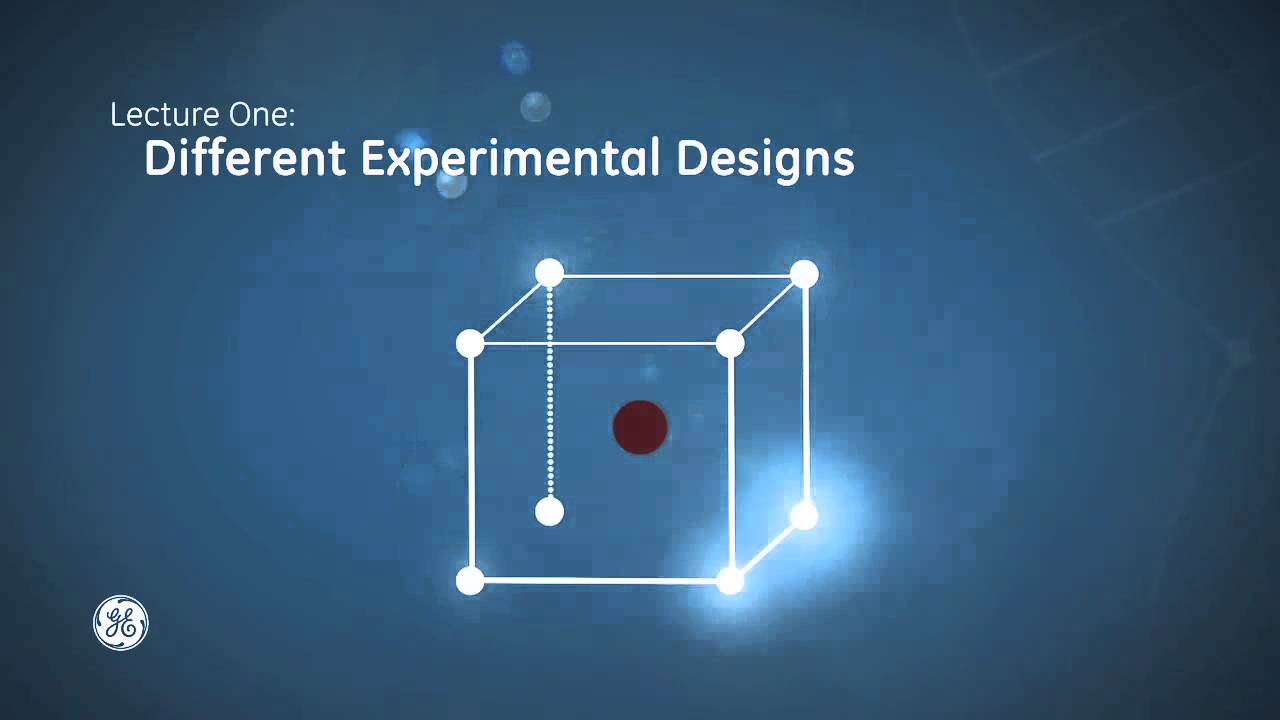
In Factorial Design, researchers are not satisfied with just studying one independent variable. Nope, they want to study two or more at the same time to see how they interact. Quasi-experiments still play with an independent variable, just like their stricter cousins.
Examples of the three types of Experimental Research Design
Optimal performance of your process is locked inside current performance, just waiting to be discovered. The optimal process can emerge once all the variables are adjusted appropriately. The Design of Experiments (DOE) tool helps align process variables and arrange them to ensure optimal performance. And while each of the highest and lowest levels for your factors may make sense in isolation, the combination may not be possible. For instance, investigating the effect of several carbon sources on bacterial growth could involve a low level or zero for each source individually. Equally, large amounts of different carbon sources could overwhelm the bacteria.
Sports Nutrition Bars Study
Double-Blind Studies in Research - Verywell Mind
Double-Blind Studies in Research.
Posted: Wed, 12 Apr 2023 07:00:00 GMT [source]
Blocking can be used to prevent experimental results being influenced by variations from batch to batch, machine to machine, day to day, or shift to shift. In the eight-run, three-factor study, for example, let's assume there was only enough thrombin enzyme in a batch for four mixes. Let's also assume any batch-to-batch difference could affect the conclusions.
To study the how leading questions on the memories of eyewitnesses leads to retroactive inference, Loftus and Palmer (1974) conducted a simple experiment consistent with true experimental design. [This blog was a favorite last year, so we thought you'd like to see it again. ].Whether you work in engineering, R&D, or a science lab, understanding the basics of experimental design can help you achieve more statistically optimal results from your experiments or improve your output quality. The key difference between observational studies and experiments is that, done correctly, an observational study will never influence the responses or behaviours of participants.
In most practical applications of experimental research designs there are several causes (X1, X2, X3). In most designs, only one of these causes is manipulated at a time. Some drugs are best delivered via a time-release schedule, dissolving slowly as they move through the body, and a special type of formulation is required to enable this. To design this type of formulation, Almac’s researchers use DOE to try out multiple different formulation ingredients (excipients), and manufacturing parameters to attain the correct drug release profile. With DOE’s help in multifactor investigation at minimal experimental runs, Almac is able to control costs for its clients as less costly raw materials are consumed in each experimental run.
So before you begin, you need to sketch out a plan for your campaign. And as with every scientific experiment, you always start by framing your question as a hypothesis. They typically employ dozens or hundreds of runs—and the variations between runs are minute. For DOEs that surpass a certain scale, you’d end up driving yourself mad trying to manually pipette 10 or more liquids into wells that are millimeters apart. In your research design, it’s important to identify potential confounding variables and plan how you will reduce their impact.
Optimize resources spent on running consumer studies
Flexible experimental designs for valid single-cell RNA-sequencing experiments allowing batch effects correction - Nature.com
Flexible experimental designs for valid single-cell RNA-sequencing experiments allowing batch effects correction.
Posted: Wed, 01 Jul 2020 07:00:00 GMT [source]
Let's say researchers are testing a new medication for high blood pressure. Participants might have different ages, weights, or pre-existing conditions that could affect the results. Now let's turn our attention to Covariate Adaptive Randomization, which you can think of as the "matchmaker" of experimental designs. In an Adaptive Design, researchers can make changes to the study as it's happening, based on early results.
How to reduce errors in Experimental Design?
If you toss ingredients into a bowl without measuring, you'll end up with a mess instead of a tasty dessert. The researcher then compared the scores on the exam for students in each condition. The results revealed that natural sunlight produced the best test scores, followed by yellow light and fluorescent light. The results indicate that participants that received the medication had significantly lower levels of heart disease than participants that received no medication. Researchers decide to conduct a study on whether men or women benefit from mindfulness the most. So, they recruit office workers in large corporations at all levels of management.
Randomization eliminates the probability of bias from the result of the experimental research design. The experiment is used for research settings where randomization is not possible. For instance, if a new drug is showing really promising results, the study might be adjusted to give more participants the new treatment instead of a placebo.
Each step helps make sure that you're setting up a fair and reliable way to find answers to your big questions. In the later part of the 20th century and into our time, computers have totally shaken things up. Researchers now use super powerful software to help design their experiments and crunch the numbers. Skinner even built boxes—called Skinner Boxes—to test how animals like pigeons and rats learn. Their work helped shape how psychologists design experiments today. Watson performed a very controversial experiment called The Little Albert experiment that helped describe behaviour through conditioning—in other words, how people learn to behave the way they do.
In planning an experiment, it is necessary to limit any bias that may be introduced by the experimental units or experimental conditions. Strategies such as randomization and blocking can be used to minimize the effect of nuisance or noise elements. Randomly allocating participants to independent variable conditions means that all participants should have an equal chance of taking part in each condition. DOE is about creating an entity of experiments that work together to map an interesting experimental region. So with DOE we can prepare a set of experiments that are optimally placed to bring back as much information as possible about how the factors are influencing the responses. Next, we evaluate what will happen when we fix the volume at 550 ml (the optimal level) and start to change the second factor.
With so many variables, it can be tough to tell which ones are really making a difference and which ones are just along for the ride. Because entire groups are assigned to each condition, there's a risk that the groups might be different in some important way that the researchers didn't account for. That's like having one sports team that's full of veterans playing against a team of rookies; the match wouldn't be fair.
No comments:
Post a Comment